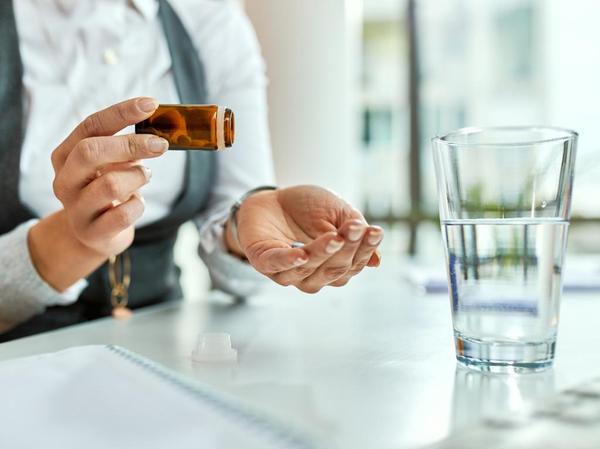
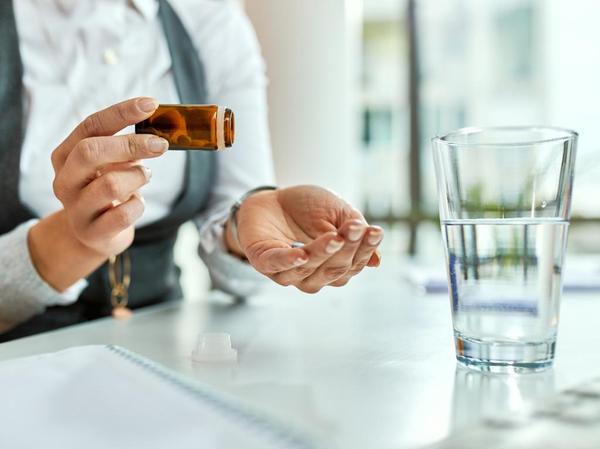
I'm a data scientist with 7 years of experience collaborating with executive-level leadership and 14+ years in quantitative fields who specializes in experimental design and custom programming that transforms complex data challenges into measurable business impact. I excel at building cross-functional relationships that uncover hidden value, using machine learning and statistical analysis to inform data-driven decision making and solve problems. I am also a STEM educator and have regularly mentored data scientists new to the field.
Penn Cobalt is a mental health platform for Penn employees. I validated the entire database to ensure the data accuracy and expected functionality of the site's diagnostic and self-help tools.
After the initial site setup, I worked with the project director to continuously improve engagement with the site through A/B testing and other statistical techniques. This included identifying user segments for specialized targeting and resulted in adding new features to the site based on testing results.
The goal of Blue Coats was to improve the well-being and financial health of the Emergency Department.
Ultimately, I identified an untapped data source and tens of thousands of dollars in monthly losses due to inefficiencies traced to a faulty supply closet scanner that was not being reviewed.
To do this, we spoke to staff directly in order to identify problems that were buried within a complex hierarchy. By combining staff knowledge with novel datasets, I quickly identified the issue after designing a data collection, processing, and storage pipeline and reviewed its outcomes. The project was so successful that the Department of Medicine purchased its own iteration of the project for the upcoming business cycle.
The goal of the project was to get a full snapshot of all of Yelp's entire healthcare database. This required that I process the raw data into validated, analysis-ready files for facilities, specific facility categories, and facility reviews. Data modalities included structured, unstructured, and geographical data points.
Over the course of six years, I maintained the project at low cost in a production environment on AWS indefinitely without a single day of data loss. I developed an automated weekly data monitoring and reporting system to ensure data quality over time.
This data served as a foundation for over twenty publications in top-tier medical journals. It also served as a high-value source of patient perceptions of care delivery for health systems looking to improve their facilities.
Data Scientist
University of Pennsylvania Healthcare System
Center for Healthcare Transformation and Innovation
Philadelphia, PA
February 2018-August 2024
Machine Learning and Data Scientist Intern
Aramark Corporation
Philadelphia, PA
May-August 2016
Physics Educator
Paul VI High School
Haddonfield, NJ
September 2010-June 2014
MS, Scientific Computing
Rutgers University–Camden
MA, Economics
Concentration in Applied Econometrics
University of Delaware
BA, Physics
University of Delaware
Programming |
Statistics |
Business Platforms |
Visualization |
Machine Learning |
Cloud Platforms |
High-Performance Computing |
NLP |
Data Platforms |